What is a Knowledge Graph?
A knowledge graph is a powerful tool for organizing and understanding information. It represents knowledge in a structured way, making it easier for machines to process and analyze.
Key Features of a Knowledge Graph:
- Entities: Real-world objects, such as people, places, things, or concepts.
- Relationships: Connections between entities, such as “is married to,” “works for,” or “is located in.”
- Properties: Attributes of entities, such as “name,” “age,” or “occupation.”
- Triples: Fundamental units of information in a knowledge graph, consisting of an entity, a relationship, and another entity or a literal value.
- Ontologies: Formal representations of a domain of knowledge, including entities, relationships, and properties. Ontologies provide a shared understanding of the domain and ensure consistency in the knowledge graph.
Benefits of Knowledge Graphs:
- Improved Information Retrieval: Knowledge graphs enable machines to understand the relationships between entities, leading to more accurate and relevant search results.
- Enhanced Question Answering: By leveraging the structured information in knowledge graphs, machines can provide comprehensive answers to complex questions.
- Personalized Recommendations: Knowledge graphs can be used to personalize recommendations for products, services, or content based on user preferences and interests.
- Fraud Detection: Analyzing patterns in knowledge graphs can help identify fraudulent activity.
- Drug Discovery: Knowledge graphs can be used to identify potential drug targets by analyzing relationships between genes, proteins, and diseases.
Data Peace Of Mind
PVML provides a secure foundation that allows you to push the boundaries.
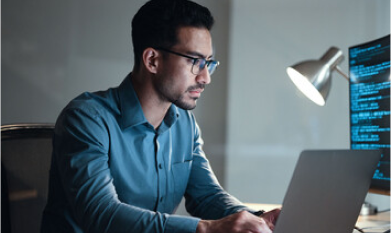
Examples of Knowledge Graphs:
- Google Knowledge Graph: A massive knowledge graph used by Google to enhance search results and provide information about entities.
- Freebase: A collaborative knowledge graph that contains information about millions of entities and relationships.
- DBpedia: A knowledge graph extracted from Wikipedia that provides information about entities and concepts related to Wikipedia articles.
Potential Use Cases for Knowledge Graphs:
- Search engines: Enhancing search results by understanding the relationships between entities.
- Question answering systems: Providing answers to complex questions based on knowledge graphs.
- Recommendation systems: Recommending products, services, or content based on user preferences and knowledge graphs.
- Fraud detection: Identifying fraudulent activity by analyzing patterns in knowledge graphs.
- Drug discovery: Identifying potential drug targets by analyzing relationships between genes, proteins, and diseases.
- Personalized learning: Adapting educational content to individual learners based on their knowledge and preferences.
- Financial analysis: Understanding relationships between companies, industries, and markets.
- Social media analysis: Identifying trends and insights from social media data.
Conclusion:
Knowledge graphs are a powerful tool for organizing and understanding information. Their ability to represent knowledge in a structured way makes them valuable for various applications, including search engines, question answering systems, recommendation systems, and more. As the field of knowledge graphs continues to evolve, we can expect to see even more innovative applications emerge in the future.