What is Differential Privacy (DP)?
Differential privacy (DP) is a method of analyzing data in a way that protects the privacy of individuals in the dataset.
It works by mathematically guaranteeing that seeing the analysis results won’t tell you anything more about any one person, even if you know whether or not they are in the data. Differential privacy is a powerful tool used in many fields, such as healthcare, finance, and social science. It allows researchers and companies to get valuable insights from data without putting people’s privacy at risk.
Differential privacy algorithms add noise to the data before it is analyzed. This noise is carefully calibrated to ensure it doesn’t distort the overall trends, but it’s enough to hide what any single person’s data contributed. This way, you can still learn useful things from the data, like how many people prefer option A or B, without being able to say precisely who chose which.
What are the benefits of differential privacy?
Differential privacy has gained significant attention in recent years due to its robust privacy guarantees and applicability across various domains. Let’s talk about the key benefits of employing differential privacy:
- Provable guarantees: Unlike anonymization techniques, which can be vulnerable to re-identification attacks, DP provides mathematically guaranteed privacy protection. This means you can quantify the level of privacy offered by the mechanism.
- Resilience to adversarial attacks: DP is strong against even sophisticated attacks where someone might try to learn information about a specific person in the data. This is because the random noise added by DP makes it difficult to link specific results back to individuals.
- Enables data sharing: DP allows collaboration and data sharing between organizations without compromising individual privacy. This is because the analysis only reveals general trends, not details about specific people.
- Plausible deniability: DP allows individuals to plausibly deny their contribution to the data. Even if someone knows the data was collected with DP, they can’t be sure their information wasn’t masked by the added noise.
- Modular design: DP algorithms can be combined to perform complex analyses while maintaining overall privacy guarantees. This makes it a flexible tool for various data analysis tasks.
Data Peace Of Mind
PVML provides a secure foundation that allows you to push the boundaries.
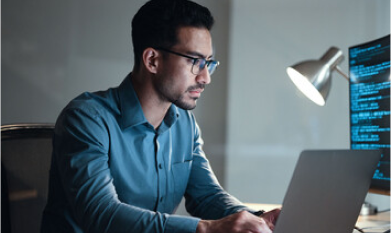
How does differential privacy work?
Differential privacy is a concept in privacy preservation that allows algorithms to release information about a dataset while preserving the privacy of individual records. The idea is to add a small amount of noise to the output of a query, such that the presence or absence of any individual’s data does not significantly affect the result.
Here’s a step-by-step explanation of how differential privacy is carried out:
- Prepare the dataset: The dataset is divided into two parts: a training set and a test set. The training set is used to train the algorithm, while the test set is used to evaluate its performance.
- Train the algorithm: The algorithm is trained on the training set. This involves learning the underlying structure of the data and any patterns that may exist.
- Apply differential privacy: Before releasing any information about the dataset, the algorithm adds a small amount of noise to the output of a query. This noise is chosen from a distribution that is similar to the distribution of the original data.
- Evaluate the algorithm: The algorithm’s performance is evaluated on the test set. This involves comparing the output of the algorithm with the actual values in the test set.
- Analyze the results: The privacy risk of the algorithm is analyzed by comparing the output of the algorithm with the actual values in the test set. If the noise added by the algorithm is small enough, it is unlikely that any individual’s data was used in the computation.
Key takeaways
DP preserves individual privacy by adding carefully calibrated noise to the data. This noise masks individual details but lets you see overall trends. This makes DP valuable in healthcare, finance, and social science. Researchers can analyze sensitive data without compromising privacy. Organizations can collaborate and share data while only revealing general trends. Individuals have plausible deniability about their contribution to the data.
The core idea is to add a tiny amount of noise to queries about the data. This noise makes it statistically impossible to tell if a specific person’s data affected the outcome. There’s a trade-off between privacy and accuracy, but DP lets you achieve both through careful noise calibration.
In summary:
- Privacy protection: Differential privacy aims to protect the privacy of individuals’ data in datasets used for analysis.
- Trade-off: There’s a trade-off between privacy and accuracy, where balancing the amount of noise added is crucial to maintaining accurate results while protecting privacy.
- Noise addition: DP intentionally adds noise to the data before performing any analysis to ensure that individual data points cannot be accurately distinguished in the output.
- Formal guarantees: DP provides formal mathematical guarantees of privacy protection, ensuring that the inclusion or exclusion of any individual’s data does not significantly affect the outcome of the analysis.
- Randomized techniques: Techniques like randomized response are often used to achieve differential privacy, where individuals respond to questions truthfully with some probability but with a chance of random flipping.
- Privacy budget: Differential privacy employs the concept of a privacy budget, representing the maximum allowable privacy loss from using DP algorithms.
- Applications: DP finds applications in various domains, including healthcare, finance, and social science research, where sensitive information needs analysis while safeguarding individual privacy.
Conclusion
It’s important to keep personal information private while still allowing researchers to analyze data.
This guide should help you better understand differential privacy and see why it’s an important tool for protecting privacy while allowing valuable data analysis.