The ability to collect, process, and derive insights from vast amounts of information is a necessity for businesses looking to stay competitive today (see also our article: “Essential Features of an Enterprise Data Platform”). As a result, developing a robust data platform strategy has become crucial for companies aiming to harness the power of their data while addressing growing concerns about privacy and security. In this article, we will explore how to build a winning data platform strategy in the current landscape, with a particular focus on how differential privacy can help address some of the most pressing challenges in data management and protection.
- What is a data platform?
- Understanding data platform strategies
- Six key components of a winning data platform strategy
- Differential privacy and data platforms
- Implementing a data platform strategy in 7 steps
- Challenges in building a data platform strategy
- A real case scenario: Netflix and Airbnb
- Conclusion
What is a data platform?
A data platform is a comprehensive system designed to collect, store, process, analyze, and manage an organization’s data assets. It serves as a centralized infrastructure that enables organizations to leverage their data for various purposes, including business intelligence, analytics, and decision-making1 (see also our article: “Essential Features of an Enterprise Data Platform”).
Here are some key features of a data platform:2
- Data integration: collecting and combining data from multiple sources (both internal and external).
- Data storage: providing scalable solutions, often including data warehouses and data lakes.
- Data processing: offering tools for cleaning, transforming, and preparing data for analysis.
- Analytics capabilities: including tools for data analysis, visualization, and reporting.
- Data governance: implementing policies and procedures to ensure data quality, security, and compliance.
- Security: incorporating robust security measures to protect sensitive information (such as differential privacy).
- Accessibility: providing controlled access to data for various users across the organization.
A well-designed data platform strategy helps organizations manage their data more effectively, derive valuable insights, and make data-driven decisions.
Understanding data platform strategies
A data platform strategy is a comprehensive plan that outlines how an organization will collect, store, process, and utilize data to achieve its business objectives. It encompasses various aspects of data management, including infrastructure, governance, analytics, and security.
There are several types of data platform strategies, each tailored to specific organizational needs:3
- Customer data platform strategy focuses on centralizing and leveraging customer data to improve marketing, sales, and customer service efforts.
- Enterprise data platform strategy aims to create a unified data ecosystem across all organizational departments and functions.
- Big data platform strategy is designed to handle and analyze large volumes of structured and unstructured data from diverse sources.
- Data management platform strategy emphasizes the organization, storage, and governance of data assets.
Regardless of the specific type, a successful data platform strategy should address the following key objectives:
- Centralize data from multiple sources.
- Ensure data quality and consistency.
- Enable efficient data processing and analysis.
- Provide secure and controlled access to data.
- Support compliance with regulatory requirements.
- Foster data-driven decision-making across the organization.
Six key components of a winning data platform strategy
As we will also see further below from real case studies, we believe that organizations should approach six key aspects to build a winning data platform strategy:4
- Data governance: Organizations should establish a robust data governance framework, including defining data ownership and stewardship roles, implementing data quality standards and processes, creating and enforcing data policies and procedures, and establishing data lineage and metadata management.
- Data integration: Organizations should create a unified view of organizational data. Key considerations include identifying and connecting diverse data sources, implementing ETL (extract, transform, load) processes, ensuring real-time or near-real-time data synchronization, and addressing data format and structure inconsistencies.
- Data storage and processing: Choosing the right data storage and processing infrastructure is critical for efficiently handling large volumes of data. Among the best options are cloud-based data warehouses and data lakes, distributed computing frameworks like Hadoop and Spark, and hybrid cloud solutions for balancing performance and cost.
- Data analytics and visualization: Empowering users to derive insights from data is a key goal of any data platform strategy. This involves implementing self-service analytics tools, developing dashboards and reporting capabilities, leveraging machine learning and AI for advanced analytics, and ensuring scalability to handle growing data volumes and user demands.
- Data security and privacy: Protecting sensitive information and ensuring compliance with data protection regulations is paramount. Key aspects include implementing robust access control mechanisms, encrypting data at rest and in transit, conducting regular security audits and vulnerability assessments, and adopting privacy-enhancing technologies like differential privacy.
- Scalability and flexibility: A winning data platform strategy must be able to adapt to changing business needs and technological advancements. This requires designing modular and extensible architectures, adopting cloud-native technologies for easy scaling, implementing APIs for seamless integration with new tools and services, and regularly reassessing and updating the strategy to align with evolving business goals.
Differential privacy and data platforms
As organizations collect and analyze increasingly large amounts of personal data, concerns about privacy and data protection have grown exponentially. Differential privacy has emerged as a powerful tool for addressing these concerns while still enabling valuable data analysis.
Differential privacy is a mathematical framework that allows organizations to share aggregate information about a dataset while withholding information about individuals within the dataset. It works by adding carefully calibrated noise to the data or query results, making it virtually impossible to determine whether any specific individual’s information was included in the dataset.
Key benefits of incorporating differential privacy into your data platform strategy include:
- Enhanced data protection: Differential privacy provides strong guarantees against re-identification attacks, even when combined with external data sources.
- Compliance with privacy regulations: Many data protection laws, such as GDPR and CCPA, require organizations to implement strong privacy safeguards. Differential privacy can help meet these requirements.
- Increased data utility: By allowing controlled access to aggregate data, differential privacy enables organizations to derive valuable insights while protecting individual privacy.
- Trust-building: Implementing differential privacy demonstrates a commitment to protecting user privacy, which can enhance trust and reputation.
- Future-proofing: As privacy regulations evolve, differential privacy provides a robust foundation for adapting to new requirements.
Data Peace Of Mind
PVML provides a secure foundation that allows you to push the boundaries.
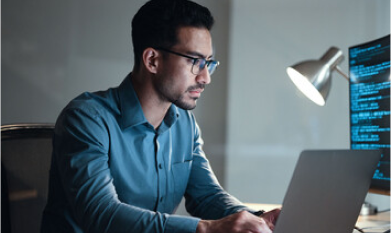
Challenges in building a data platform strategy
Some key challenges in building a data platform strategy include:5
- Security and data protection: Data security is ranked as a top challenge, with organizations needing to implement robust data governance practices and safeguard sensitive data as it’s distributed to users.6
- Operational complexity: Managing and integrating different data sources, technologies, and processes requires specialized knowledge and can be complex.7
- Compliance and regulatory requirements: Ensuring data is managed in compliance with regulations is crucial but challenging.8
- Skill shortages: There’s often a lack of internal capability and specialized skills needed to implement and manage modern data platforms.9
- Cost and budget constraints: Data platforms can have expensive run costs and require costly specialist resources.10
- Scalability: Designing a platform that can scale to meet growing data needs and evolving requirements.11
- Technology selection: Choosing the right technologies that align with organizational needs, existing systems, and future goals.
- Data volume and diversity: Many organizations struggle with the increasing volume and diversity of data.12
- Data availability and quality: Ensuring data is readily available in the right format and of sufficient quality.
To address these challenges, organizations need to carefully consider factors like their specific use cases, internal capabilities, budget, technology orientation, and data volumes when designing their data platform strategy. It’s also important to start with a minimally viable solution that addresses critical needs and can scale over time.13
Implementing a data platform strategy in 7 steps
Implementing a winning strategy requires a structured approach and ongoing commitment. Some of the key steps are summarized below:14
- Assessment: Organizations should begin by assessing their current state, including existing data assets, systems, and team capabilities. This evaluation helps identify gaps and areas for improvement.
- Define objectives: Organizations should define clear, SMART objectives that align with their overall business goals and prioritize initiatives based on their potential impact and feasibility.
- Build teams: Organizations should build a cross-functional team with diverse skills and ensure executive support.
- Select the right technologies: Organizations should carefully select technologies that fit their strategy, considering factors like scalability, integration capabilities, and support for privacy-enhancing technologies.
- Stages implementation: Organizations should implement their strategy in phases, starting with a pilot project to demonstrate value. Gradually expand the scope, focusing on high-priority use cases and continuously iterating based on feedback.
- Train: Organizations shall provide comprehensive training and ongoing support to users at all levels. Establish a center of excellence to share best practices and drive innovation.
- Monitor: Organizations should monitor progress using established KPIs and regularly assess the impact of their strategy on business outcomes.
This phased, flexible approach allows organizations to implement their data platform strategy effectively, balancing immediate needs with long-term goals while fostering a culture of continuous improvement.
A real business scenario: Netflix and Airbnb15
In the rapidly evolving digital landscape, companies like Airbnb and Netflix have set benchmarks in leveraging data to drive business growth and enhance user experiences. Their data platform strategies are sophisticated, integrating advanced analytics, machine learning, and robust data governance to stay ahead of the competition.
Airbnb uses data to enhance user experiences and optimize operations. Key elements of their strategy include:
- User search history: analyzing search patterns to personalize results.
- User ratings and reviews: using feedback to rank listings.
- Location-based data: tailoring search results based on user location.
- Predictive analytics: anticipating user preferences to improve recommendations.
- Data-driven decision making: using data to inform product design and marketing strategies.
Airbnb’s data platform supports over 100 million users and 2 million listings, requiring a scalable and robust infrastructure. To handle large data volumes efficiently, Airbnb uses cloud-native technologies and a microservices architecture.
Netflix leverages data to deliver personalized content and optimize streaming quality. Key elements of their strategy include:
- User behavior analysis: tracking viewing habits to recommend content.
- Content performance metrics: analyzing how different shows and movies perform.
- Real-time data processing: using tools like Apache Kafka and Spark for real-time analytics.
- Machine learning models: predicting user preferences and optimizing content delivery.
Netflix captures roughly 500 billion events per day, which translates to 1.3 petabytes of data. Its data architecture includes cloud-based storage, distributed computing frameworks, and advanced analytics tools.
When looking at how these two companies have developed their sophisticated data platform strategies, they have successfully balanced innovation with privacy protection. Their strategies are designed to handle vast amounts of data efficiently and securely while driving business growth and enhancing user experiences.
The key components of their approaches include the areas mentioned above, such as robust data governance frameworks, advanced data integration techniques, and scalable cloud-based storage and processing solutions. They leverage cutting-edge analytics and visualization tools, including machine learning and AI, to derive actionable insights from their data.16
A critical aspect of their strategies is the implementation of strong data security measures and privacy-enhancing technologies, such as differential privacy.17 This allows them to protect individual user privacy while still extracting valuable aggregate insights. Their architectures are designed to be modular, scalable, and flexible, enabling continuous improvement and adaptation to changing business needs.18
By effectively balancing data utility with privacy protection, these two companies demonstrate how organizations can leverage data to drive success while building customer trust in an increasingly data-driven world.19
Conclusion
Building a winning data platform strategy in today’s landscape requires a delicate balance between innovation and privacy protection. By incorporating differential privacy and other privacy-enhancing technologies, organizations can create a robust foundation for data-driven decision-making while safeguarding sensitive information. As we have stressed in our previous article, “Essential Features of an Enterprise Data Platform,” a successful data platform strategy is an ongoing process of refinement and adaptation.
By following the guidelines outlined in this post and maintaining a commitment to both data-driven innovation and privacy protection, organizations can develop a data platform strategy that drives business success while building trust with customers and stakeholders. This balanced approach is the key to staying competitive and responsible today and for years to come.
2 Pivotl, “Five Points To Help You Avoid Choosing The Wrong Data Platform,” https://www.bepivotl.com/insights/avoid-choosing-the-wrong-data-platform-solution/
3 See Note 2
4 James Green, “7 Rules of Creating a Successful Data Platform,” 19 January 2021, Ancoris, https://www.ancoris.com/blog/7-rules-creating-successful-modern-data-platform
5 James Watson, “What are the Challenges of Building a Data Platform,” 14 Narch 2023, Oakland, https://weareoakland.com/blog/what-are-the-challenges-of-building-a-data-platform/
6 Stephen Catanzano, “Security a Top Challenge in Building a Modern Data Platform,” 12 September 2023, Tech Target, https://www.techtarget.com/searchdatamanagement/opinion/Security-a-top-challenge-in-building-a-modern-data-platform
7 See Note 2
8 See Note 2
9 See Note 2
10 See Note 2
11 See Note 2
12 See Note 2
13 Madiha Kahlid, “Left Shift Data Platform,” 29 February 2024, Maddyness, https://www.maddyness.com/uk/2024/02/29/left-shift-data-platform-how-to-overcome-early-stage-startup-challenges-to-be-data-driven/
14 Adrian Both et al, “Building a Great Data Platform,”, 2018, McKinsey, https://www.mckinsey.com/~/media/McKinsey/Industries/Electric%20Power%20and%20Natural%20Gas/Our%20Insights/Building%20a%20great%20data%20platform/Building-a-great-data-platform-final.pdf
15 Jasmine Bilham, “Data Analytics,” 10 March 2023, Raw Data, https://raw.studio/blog/data-analytics-airbnb-netflix-amazon-spotify/
16 Zymr, “this-is-how-innovators-like-airbnb-netflix-and-uber-have-turbocharged-their-business-growth,” 28 May 2024, https://www.zymr.com/blog/this-is-how-innovators-like-airbnb-netflix-and-uber-have-turbocharged-their-business-growth
17 Akshaya Mani, “balancing-data-utility-and-privacy-how-differential-privacy-benefits-media-companies,” 1 December 2023, Optable, https://www.optable.co/post/balancing-data-utility-and-privacy-how-differential-privacy-benefits-media-companies
18 See Note 16
19 See Note 16