In the current data-driven world, there is a huge demand for high-quality data. However, obtaining such data is not always feasible due to privacy concerns, data scarcity, or costs associated with its collection. As a solution, synthetic data has come into place.
What is Synthetic Data?
Synthetic data refers to artificially generated data that imitates the statistical properties of real-world data.
Real data is collected from observations or measurements, while synthetic data is created using algorithms, models, or simulations.
Synthetic data provides a substitute for real data in situations where obtaining or sharing real data is impractical, costly, or ethically problematic.
How Do You Generate Synthetic Data?
The process of generating synthetic data will consist of the following steps.
- Real data collection: This step will include gathering real-world data from various sources, such as databases, APIs, or data providers.
- Data cleaning and harmonization: Collected data must be processed and cleaned. This includes handling missing values, removing duplicates, correcting errors, and standardizing formats.
- Data privacy evaluation: This step will evaluate the privacy implications of the real data. It will identify any sensitive or personally identifiable information (PII) in the data. There shouldn’t be any privacy risks.
- Synthetic data generation model: In this step, a data generation model or algorithm is designed. This model should be capable of creating synthetic data that resembles the statistical properties and patterns observed in the real data.
- Data generation process: In this step, the data generation model will be used to generate the synthetic data. The model should create artificial examples that simulate the characteristics and distribution patterns of the real data.
- Data utility evaluation: In this step, the quality and usefulness of the data will be assessed. The synthetic data will be compared with the real data to evaluate how well it captures essential patterns, features, and statistical properties. You can use evaluation metrics to assess the utility of the synthetic data.
- Iterative refinement: In this step, we will adjust the data generation model or algorithm based on the feedback from the evaluation or iterate through the process to improve the quality and fidelity of the synthetic data.
Applications of Synthetic Data
The applications of synthetic data spread across various domains. Here’s how we can apply synthetic data.
- Research and development: Synthetic data enables experimentation and exploration of hypotheses without constraints imposed by limited or sensitive real-world data. Therefore, it provides access to diverse datasets for analysis and testing.
- Testing and validation: Synthetic data supports the development and validation of algorithms, models, and systems in various domains.
- Training machine learning models: Synthetic data can be used to augment existing datasets or generate new ones to diversify training samples, improving model performance and generalization.
- Privacy-preserving data sharing: Synthetic data facilitates data sharing and collaboration without compromising individual privacy or sensitive information. It also enables researchers, organizations, and institutions to exchange data for analysis and research purposes while protecting confidentiality.
Use cases
As depicted in the diagram, synthetic data can be used in these areas:
- Healthcare
- Agriculture
- Banking & Finance
- E-commerce
- Manufacturing
- Disaster Prediction and Risk Management
- Automotive and Robotics
Data Peace Of Mind
PVML provides a secure foundation that allows you to push the boundaries.
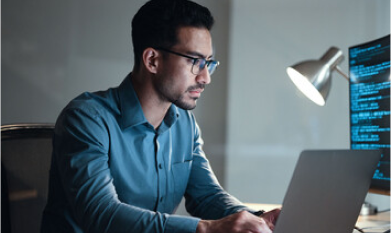
Advantages and Challenges
The adoption of synthetic data offers several advantages, but it also poses some challenges. Some of the advantages and challenges are listed below.
Advantages
- Privacy preservation: Synthetic data eliminates privacy concerns by generating data that contains no identifiable information.
- Cost-effectiveness: It reduces the need for extensive data collection efforts or expensive acquisition of proprietary datasets.
- Diverse data generation: Synthetic data allows for the creation of diverse datasets, augmenting existing data or generating entirely new ones.
Challenges
- Accuracy and realism: Ensuring synthetic data accurately reflects the characteristics of real-world data can be challenging.
- Bias and generalization: Mitigating bias and ensuring the generalizability of models trained on synthetic data require careful consideration and validation strategies.
- Validation: Rigorous validation processes are necessary to ensure the quality and fidelity of synthetic data, as inaccuracies may impact the reliability of research outcomes.
- Adoption hurdles: Despite its potential benefits, the adoption of synthetic data may face resistance due to skepticism or unfamiliarity with the concept among stakeholders.
Best Practices for Using Synthetic Data
Even though the application of synthetic data can be challenging, the use of below best practices can mitigate those challenges.
1. Quality assessment
- Conduct thorough assessments to ensure the accuracy of synthetic datasets.
- Validate synthetic data against real-world data to verify its consistency and reliability.
- Employ appropriate metrics and evaluation techniques to measure the quality and performance of synthetic data.
2. Ethical considerations
- Adhere to ethical guidelines and principles when generating, using, and sharing synthetic data.
- Ensure transparency and accountability in the generation process, disclosing any limitations or biases.
- Respect privacy rights and confidentiality.
3. Validation of model performance
- Evaluate the performance of machine learning models trained on synthetic data against real-world benchmarks.
- Conduct strict validation experiments to assess the robustness of models.
- Introduce cross-validation techniques and sensitivity analyses to identify potential biases or shortcomings.
Wrapping Up
The introduction of synthetic data has changed the way we approach data generation, utilization, and privacy preservation.
Synthetic data can be used by researchers, businesses, and policymakers to unlock insights, drive innovation, and protect individual privacy. Its ability to mimic the statistical properties of real-world data proves useful as a tool for data-driven decision-making.
Even though there are challenges in using synthetic data, with the use of best practices, we can make the most out of it.
As we continue to explore its potential and overcome challenges, synthetic data will act as a revolutionary way to interact with data in the digital age.
Embracing synthetic data alongside real data will pave the way for a more dynamic, inclusive, and responsible approach to data utilization and analysis.